How Machine Learning Can Improve Recruiting
The 10 Most Influential Leaders in Talent Acquisition Industry 2022
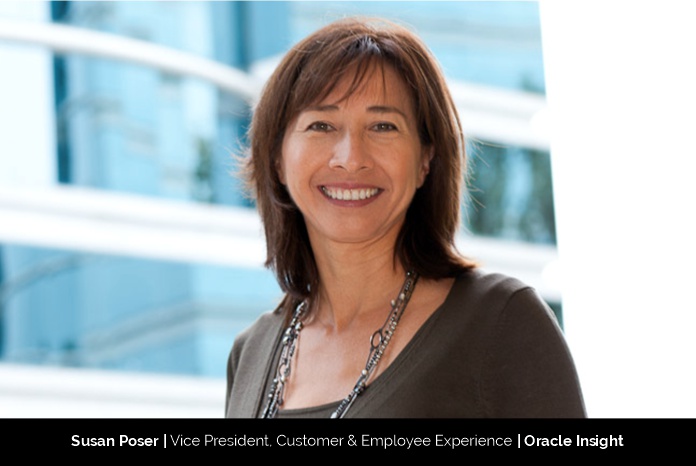
With the right strategy, computers can
find correlations that humans overlook, leading to better candidates.
How do you know you are hiring the right
candidates? According to the Society for Human Resource Management, the average
cost per hire is US$4,129, and it takes an average of 42 days to fill an open
requisition. Imagine that amount being multiplied by all of your open positions
over the course of the year. Then there is the issue on the other side of the
coin—what if you rush to hire and you don’t hire the right person? A study
found that 41% of employers estimated a single bad hire costs US$25,000, and 25%
put the figure at US$50,000 or more.
What can companies do to reduce the cost
per hire, as well as to reduce the chance of a bad hire? One approach is to use
machine learning. Today, machine learning is already being used to make
recruiting more efficient in three different areas:
- Application and résumé review: Screening résumés based on
keywords, leveraging social data to identify candidates, and using online
questionnaires
- Pre-engagement: Deploying artificial intelligence (AI)
assistants and chatbots to respond to candidate inquiries or schedule
interviews
- Talent sourcing: Narrowing top candidates from a large pool
using key attributes
How is machine learning doing this? Machine
learning iteratively applies algorithmic analytical models to preprocessed data
to uncover hidden patterns or trends that can be used to flag ideal résumés to
review, predict the correct response to inquiries in the pre-engagement, or
identify the best candidates for talent sourcing. While all of these areas can
help reduce time and money spent to fill the position, there is one we believe
can make the biggest impact to ensure you are hiring the right person: talent
sourcing.
It is critical to eliminate the bias
that could become inherent in machine learning.”
Will machines have better success finding
the right candidates for your open positions than your recruiters? Ideally, a
computer will find correlations and patterns that you would overlook, which
would lead to increasingly higher-quality candidates. Here are some
considerations if you are thinking about using machine learning to help with
talent sourcing.
Passive Sourcing
To leverage machine learning, you need to
first define the variables on which to “train” the system. The variables you
should consider will depend on your approach. Are you sourcing passive
candidates—people who aren’t actually looking for a new job—or are you looking
to narrow top candidates from a large pool of applicants? If you are doing the
former, you might want to consider attributes such as how recently or
frequently they have updated their LinkedIn profile, because this could
indicate that they might start looking for a job or are already on the hunt. Or
consider factors affecting current employer stability (such as mergers and
acquisitions, layoffs, and stock fluctuations). You could also look at market
indicators to help predict a downturn in a particular industry or company,
which might create a plethora of available candidates, giving you an early
advantage.
Active Sourcing
On the active recruiting side, imagine
receiving hundreds, if not thousands, of applications for open positions. Here
is where machine learning can help narrow the top candidates, depending on the
trainability of your data. Do you have enough historical and relevant data on
successful candidates or employees to train your system? It’s as though you are
looking for a “mini-me” based on the profile of the “ideal” employee. The
attributes you consider here will depend on the role, but one approach would be
to reverse-engineer the best fit by looking at the attributes of successful
employees in that role, such as their work experience, industry, and work
product. Other attributes to consider would be the number of jobs they’ve had
in the last five years, their tenure in each job, major and extracurricular
college activities for a recent-college-graduate hire, or hobbies (competitive
sports might be good for a sales role). You could also leverage machine
learning to target candidates who have a higher probability of success based on
prior recruiting strategies.
This article was originally published
on Profit online (https://blogs.oracle.com/profit/).