How is Deep learning benefiting healthcare?
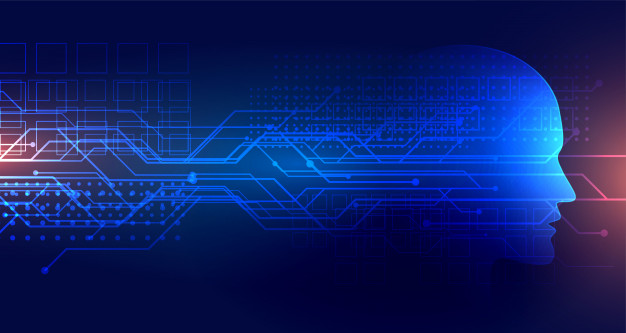
Deep learning is a subgroup of machine learning, which is again
a part of the larger technological advancement called Artificial intelligence
(AI). While artificial intelligence deals in making machines as
intelligent as humans, it looks into networks that learn from unstructured data
independently.
Deep learning has been a boon to the field of healthcare as
it is known to provide the healthcare industry with the ability to analyze data
at exceptional speeds no matter the size without compromising
on accuracy, which mostly suffered due to human errors earlier. Even though not
a long time has gone by, this method is already making its mark in medical imaging
solutions. There are chatbots that have identified patterns in thsymptoms
presented by the patients. Certain algorithms are now capable of
identifying types of cancers and certain rare diseases.
Benefits of Deep learning
A sleek, elegant, and smart blend of both machine learning
and AI, learning uses a layered algorithmic architecture to sift through data
at a remarkable rate. With the amount of labor that it provides with such
efficiency, healthcare organizations of all sizes, types, and specialties over
time have considered this marvel to be the pioneer of futuristic medical
possibilities. They are all the while becoming increasingly interested in how
artificial intelligence can support better patient care while dramatically
cutting down costs and improving efficiencies.
Some of the benefits
of in healthcare that have made it an undeniable aspect in modern
times are stated below:
The power to analyze medical history: A blessing in its entirety,there is a lot
of unstructured data almost every day in large numbers in the form of patient
records, past treatments, and the medical history of the patient’s family. All
of which has been always known to have faced some sort of human error while
being drafted. This branch of machine learning discerns all such medical
concerns as they help is an accurate analysis of the historical data of
patients and even extend a helping hand as far as predicting accurate conditions
about their health by extracting insights from past data like diseases, family
background, and hereditary diseases and so on.
Administrative task management: Learning in depth has taken up amyriad of tasks in order to facilitate more concentration and funding on providing the best possible healthcare to the people across the globe. It enables healthcare professionals to automate a lot of administrative tasks so the complete focus is centered on serving better rather than diversified stands to exempt any sort of human error. From rescheduling appointments to filing the appointment records to sending suggestions to patients and forwarding emergency calls, the list of tasks that can be performed are endless.
Identifying insurance fraud: Insurance companies are now using deep learning
for figuring out medical insurance fraud claims. This has been
achieved by automating fraud detection and using predictive intelligence inpre-identifying
a future fraud. US healthcare program Medicare, which is fundedby the Federal
government, is responsible for providing health insurance to those who are 65
years and above. This insurance is also available for
individuals with permanent disabilities. There is a lot of loss of billions of tax
payers’ dollars due to misuse, fraud, wastage and abuse. Medicare reports a loss
between $21 billion to $71 billion dollars per year to fraud, wastage, misuse
and abuse. Manually auditing every insurance claim is impossible. Here
is where deep learning steps in to identify and control medical insurance fraud.
Detection and classification of Alzheimer’s: recently, deep learning has gained a lot of attention in early detection and classification of Alzheimer’s disease. This has been possible due to the large scale analysis of neuroimaging data. As we know, Alzheimer’s is the most common type of dementia and is the 6th leading cause of death in the US. Dealing with thisdisease cost $277 billion in 2018 in the US alone. Since it is an irreversible disease, early detection will allow earlier medication to prevent the progression in a patient
Genomics: This subset of machine learning has a bright future in understanding
genomes. Genomics is the study of understanding elements of genetics. In a
human being the genome is made up of over 3 billion pairs. Reading the entire
information enwrapped in a DNA is complex and impossible to
do using traditional methods. Deep learning’s expertise in large amounts of data
crunching will be crucial in future breakthrough in genomics.
Conclusion
Deep learning has a promising future in healthcare especially in detecting early stages of cancer, its diagnosis and treatment. It also looks promising when it comes to epidemiology, public health, clinical genetics, crop improvement,functional genomics and phylogenetic analyses